Doctor Chatbot: The EUʼs Regulatory Prescription for Generative Medical AI
Doctoral Research Fellow
Faculty of Law, UiT the Arctic University of Norway. Norwegian Centre for Clinical Artificial Intelligence, University Hospital of Northern Norway.mathias.k.hauglid@uit.noProfessor
Norwegian Research Center for Computers and Law, University of Oslotobias.mahler@jus.uio.noPublisert 30.06.2023, Oslo Law Review 2023/1, side 1-23
This article analyses the EUʼs regulation of medical artificial intelligence (AI) from a product safety perspective, concentrating on the interplay between the proposed AI Act (AIA) and the Medical Device Regulation (MDR). Recent advances in AI development illustrate the future potential of generative AI technologies, including those based on Large Language Models (LLMs). In a medical context, AI systems with different degrees of generativity are conceivable. These AI systems can pose new types of risks that are specific to AI technologies, as well as more traditional risks that are typical of medical devices. The proposed AIA is intended to address AI-specific risks foreseen by the EU legislature, whereas the MDR addresses more traditional medical risks. Through two case studies which display different degrees of generativity, this article identifies regulatory lacunae in the intersection between the AIA and the MDR. The article suggests that the emerging regulatory framework for medical AI systems potentially leaves certain AI-specific risks as well as certain typical medical device risks unregulated. Finally, the article discusses possible solutions that are compatible with the intentions of the EU legislature pertaining to the regulation of medical AI systems.
Keywords
- AI Act
- medical devices
- generative artificial intelligence
- chatbots
- large language models
1. Introduction
In recent years, the development and use of technologies involving artificial intelligence (AI) has proliferated in the EU and internationally. In the health sector, the increased use of AI raises several legal and policy issues. With this article, we contribute to the discussion on the EUʼs regulation of medical AI from a product safety perspective. We focus on the EUʼs emerging product safety framework as it will exist after the future introduction of the proposed EU Artificial Intelligence Act (AIA). In the context of medical AI systems, there will be considerable interplay between the AIA and the EUʼs Medical Device Regulation (MDR).
The main purpose of this article is to search for regulatory lacunae that may exist in the space between the AIA proposal and the MDR in respect of medical AI systems. Because of the way in which the two legal frameworks are interconnected, we focus on potential lacunae stemming from the two risk classification schemes for medical AI systems, respectively included in the AIA and in the MDR. While others have also argued that there may exist lacunae in the future EU legal framework, consisting of risks that are not sufficiently managed when the two laws are read in conjunction, we rely on two specific case studies to demonstrate the problem and propose solutions.
We use the term ʻmedical AI systemsʼ to refer to health-related AI systems, regardless of whether they fall under the scope of the MDR. Like other products with medical purposes, AI systems may harm patients if they do not function as intended, or if they are developed or used without appropriate safeguards. Some emerging applications of AI for health may present similar safety risks as non-AI products, while other applications may pose risks that are more specific to AI-based products. The goal of the AIA is primarily to mitigate the latter category of risks, as it is assumed that the existing product safety framework can handle risks that are similar to those posed by non-AI products. If there are risks related to AI products that are not addressed either by the existing product safety framework (especially the MDR) or by the AIA, those risks constitute what we regard as regulatory lacunae for the purposes of this article.
While the AIA considers a broader range of technologies as ʻartificial intelligenceʼ, this article primarily contemplates generative AI systems employing technologies such as machine learning (ML) methods, including deep learning, as well as natural language processing (NLP) techniques. Of particular interest is the NLP-based subset of generative Large Language Models (LLMs). When referring to generative AI, or simply AI, we allude to the various technologies mentioned above.
This article is structured as follows. Section 2 introduces the prospects and risks of medical AI systems, exemplified by recent advances in generative LLMs. Section 3 presents an overview of the emerging regulatory framework for medical AI systems, which represents the necessary background for the present article. Subsequently, Sections 4 and 5 narrow the focus on the proposed AI Act and the Medical Device Regulation. Both laws present a form of risk classification, as explained in Section 6, but this implies the possibility that some risks remain unaddressed in the intersection between the two laws. We therefore examine the potential for lacunae between the two acts in two case studies. Section 7 addresses the case of therapeutic chatbots. These systems, potentially falling into the minimal risk class, do not need to comply with the safety requirement of the AIA, even though they arguably represent examples of relatively risky medical AI systems. Section 8 addresses the case of an automated documentation system for electronic health records, which potentially may not be subject to the main AIA requirements since its classification as a ʻmedical deviceʼ is uncertain. Section 9 discusses implications of the proposed case studies and engages with existing proposals of how to address the risks of medical AI systems. Section 10 proposes solutions that we find to be compatible with the intentions of the EU legislature when it comes to the regulation of medical AI systems.
2. The Prospects and Risks of Generative Medical AI Systems
AI systems are already being used to some extent in the health sector and are expected to be used more widely in the near future. AI can be used to support clinical tasks such as diagnoses, treatment recommendations, patient monitoring, and risk assessments, as well as administrative tasks related to, eg, resource management, or to automate certain workflows or procedures, such as surgical procedures. Moreover, AI systems may be used to monitor, assess, or treat patients remotely through mobile and wearable devices.
Recent advances in AI development particularly illustrate the future potential of generative AI technologies. The term ʻgenerative AIʼ refers to AI models that are designed to generate or create new content, such as images, video, or text, that is similar to the training data the models have been exposed to. For example, the development of Large Language Models (LLMs) has enabled the creation of advanced chatbots such as ChatGPT. Because systems like ChatGPT are optimised for dialogue, they can respond to conversational prompts and adapt their responses to new input. They can produce answers that seem coherent and thoughtful, despite the fact that these systems cannot ʻthinkʼ in a traditional sense. The conversational capabilities of current LLMs provide a preliminary indication of the prospects of future generative medical AI systems. It is not inconceivable that conversational chatbots could be used to treat certain types of mental illness, mimicking conversations with a therapist. As such, chatbots could act as virtual therapists and make therapy – a limited resource – more easily accessible to patients. In this case, it would be important to ensure that the system responds adequately to problems, such as indications of suicide risk, or that it does not suggest the consumption of alcohol to treat depression. Risks related to therapeutic chatbots are further discussed in section 7.
Different applications may expose patients to different kinds of risk. This article further discusses how risks related to medical AI systems with different degrees of generativity can be mitigated through EU regulatory measures. The wider legal context for such measures can be found either in medical law, AI law or in a combination of both fields. This article focuses especially on the intersection of AI and medical law, since certain risks associated with medical AI systems may slip through the gaps between two key legal instruments, the AIA and the MDR.
3. The Emerging EU Regulatory Framework for Medical AI Systems
The use of medical AI systems can trigger, in principle, a variety of legal rules, including those related to the processing of personal data in the EU General Data Protection Regulation (and supplementing national laws), or rules governing the provision of healthcare, which are typically found in national law. In addition, the introduction of AI systems on the European market must comply with current and upcoming safety rules, which are the main focus of this article. For certain types of products which are deemed to present risks to the health and safety of EU citizens, access to the common market is contingent on conformity with harmonised safety and performance requirements laid down in EU law.
The purpose of product safety legislation at the EU level is twofold: to ensure a high level of protection from health and safety risks, and to facilitate innovation and free trade of products across EU member states. These legislative aims are equally important. Therefore, the substantive requirements to which products need to conform, as well as the procedures through which conformity may be demonstrated (conformity assessment procedures), are designed with the intent of striking an appropriate balance between them. To determine what the appropriate balance is, the EU legislature relies on risk assessments. Through risk assessments, the legislature anticipates health and safety risks associated with a category of products (eg toys, lifts, radio equipment, medical devices and soon also AI systems). Each product category is regulated according to the anticipated types of risk. For instance, toy manufacturers are obligated to consider and address elements such as a toyʼs physical attributes (eg its sharpness and the possibility of it obstructing a childʼs airway), its flammability and its toxicity. Medical AI systems can imply risks from different spheres, including risks typical to the medical context (eg misdiagnosis), as well as typical AI risks (eg automation bias). These risk types are addressed in current and proposed EU laws.
Certain medical AI systems will be classified as medical devices and thus be regulated under the MDR, particularly when they are incorporated within a physical medical device. For instance, if an AI system is incorporated into a pacemaker, the pacemaker is the medical device, and the AI system is an integrated component of the pacemaker. However, the focus of this article is on ʻstandaloneʼ medical AI systems, ie medical AI systems that serve their purpose without being incorporated into another medical device. Such a ʻstandaloneʼ AI system may in itself constitute a medical device under the MDR. The term ʻAI medical devicesʼ is used in this article to denote standalone AI systems that constitute medical devices.
Medical devices generally come in many different shapes and are used for a variety of tasks. Because of their build and the use for which they are intended, some devices are more dangerous (risky) than others. The MDRʼs risk classification scheme is meant to ensure that more risky devices are subject to heavier scrutiny before they can be placed on the common market. For less risky devices, the path to the common market is easier. This is how the EU strikes a balance between the dual aims of facilitating innovation in the medical space and protecting citizens from unjustified health and safety risks. Therefore, the risk classification schemes – for medical devices and for other product categories – are crucial to the functioning of the common market. When new technologies emerge – potentially presenting other types of risk than those anticipated by the existing legislative risk assessments – the ability of existing risk classification schemes to ensure a well-functioning marketplace may be challenged.
The EU Commission has proposed a common European framework for artificial intelligence (the AIA). The proposed AIA lays down requirements that AI systems and the ʻprovidersʼ of AI systems (in various sectors) must satisfy before the systems can be placed on the common market. In this sense, the proposal appears as a product safety act for AI systems, and applies on top of the already existing sector-specific product requirements that follow from the EUʼs product safety framework (the ʻNew Legislative Frameworkʼ/ʼNLFʼ), including the MDR. In addition to addressing safety aspects of AI systems, the AIA aims to ensure that AI systems respect existing laws on fundamental rights and Union values and to enhance the effective enforcement of fundamental rights. The EU Commission has made it clear that it only intends to impose new rules for AI to the extent that is necessary to protect EU citizens from AI-related risks in so far as those risks are not already addressed in existing EU legislation. Thus, the AIA is meant to address the specific risks posed by AI systems. These risks are typically not addressed by the existing legal framework, which focuses on more traditional products and their related risks.
To ensure that the scope of the AIA is adapted to the risks posed by AI systems, the Commissionʼs proposal relies on a preparatory risk assessment. Based on this legislative risk assessment, the Commissionʼs proposal prohibits certain AI systems because the risks associated with these systems are deemed to be unacceptable. Further, the proposed AIA sets out classification rules for distinguishing between ʻhigh-riskʼ and non-ʻhigh-riskʼ AI systems. The classification determines the requirements that will apply to the AI systems. While ʻhigh-riskʼ AI systems are subject to a set of material requirements in Title III of the proposed AIA, AI systems classified as lower risk will only be subject to minimal transparency requirements or not be regulated by the AIA. In practice, this means that the most important requirements of the AIA only apply to AI systems that are classified as ʻhigh-riskʼ. Therefore, it is crucial to determine the risk classification of any AI system, including medical AI systems, in order then to determine whether they reach the ʻhigh-riskʼ threshold. If the threshold is reached, the AIAʼs requirements apply, in addition to any other requirements, for example those included in the MDR. If the threshold is not reached, the respective AI system does not need to conform to the main requirements of the AIA. This is where the AIA is linked to the MDR and its risk classification scheme for medical devices.
As mentioned above, medical devices are also placed in different risk classes under the MDR according to a set of risk classification rules. The AIA relies on this risk classification scheme in order to determine whether additional requirements (ie those that are found in the AIA) apply. Although the details are slightly more complicated, as elaborated below, the AIAʼs requirements essentially apply in addition to the requirements of the MDR, if a medical AI system reaches a specific risk threshold in the MDR. On the flip side, the AIAʼs requirements are not applicable if a medical AI system is not classified as a medical device at all, or if it is classified in a risk class below a specific threshold. This implies the possibility that some medical AI systems might not be subject to the AIAʼs requirements. This is not necessarily a problem as long as these systems do not imply a high level of AI-specific risks.
4. ʻHigh-Riskʼ AI systems in the Artificial Intelligence Act
In the following, we concentrate on the requirements for ʻhigh-riskʼ AI systems in the AIA. This means that we disregard, inter alia, the prohibited AI systems, as it is unlikely that many medical AI systems will fall into this category, although the possibility cannot be completely excluded. This article is primarily interested in understanding the delimitation between ʻhigh-riskʼ AI systems, which are subject to specific requirements, and non-ʻhigh-riskʼ AI systems, which remain essentially unregulated by the AIA. Many AI systems do not reach the threshold of ʻhigh-riskʼ and are therefore not subject to the AIAʼs rules for ʻhigh-riskʼ AI systems. For example, a system to detect spam in an email inbox is not considered sufficiently risky to justify stringent requirements.
Title III of the proposed AIA sets out the requirements aimed at AI systems classified as ʻhigh-riskʼ. Providers of ʻhigh-riskʼ AI systems must establish a risk management system and a quality management system, implement data governance measures including an examination in view of possible biases, and draw up technical documentation. Furthermore, the ʻhigh-riskʼ AI system and the training data used to develop the system must comply with the requirements concerning the statistical properties of the data, technical documentation, record-keeping functionalities, system transparency, information to users, human oversight functionalities and requirements relating to accuracy, robustness and cybersecurity. Finally, the provider must ensure that AI systems undergo the relevant conformity assessment procedure. Having demonstrated compliance, the provider shall draw up an EU declaration of conformity and affix the CE marking of conformity.
Because the Title III requirements in the AIA do not apply to non-ʻhigh-riskʼ AI systems, there will be a significant difference in the material requirements that are applicable to ʻhigh-riskʼ AI systems and other AI systems. It follows that the role of the AIAʼs risk classification scheme is to distinguish between AI systems on which it is justified to impose the material requirements, and AI systems that ought to be allowed market access without adhering to those requirements, because they present less risk. The risk classification scheme is how the AIA strikes a balance between the two foundational values of the traditional common market for products: health/safety and innovation/trade.
Although this could be further discussed, this article assumes that the AIA works well if it regulates an AI system that in fact presents risks that justify regulation. This leaves open the possibility of two types of errors. This article does not further address the possibility that some AI systems are regulated under the AIA despite the fact that they do not present sufficient risks. Instead, we concentrate on the other type of error, ie the possibility that a medical AI system remains unregulated even though it should be, because it is risky. This means that we address the possibility of a mismatch, or lacuna, in which a medical AI system is not classified as ʻhigh-riskʼ in the AIA, despite the system implying risks that ought to be managed by applying the requirements of the AIA. With this focus in mind, we now turn to the mechanism for classifying ʻhigh-riskʼ AI systems in the AIA.
There are two ways through which an AI system can fall into the AIAʼs ʻhigh-riskʼ category. First, Annex III in the proposed AIA lists AI systems that shall be considered as ʻhigh-riskʼ. Most systems in this list are of limited relevance to medical AI, as they concentrate on contexts such as employment, law enforcement, the administration of justice, etc. Annex III focuses on specific purposes for AI systems that are classified as ʻhigh-riskʼ. In addition, in light of the discussions within the European Council and Parliament, it is anticipated that the AIA will take into account the significance of general-purpose AI systems, such as LLMs not intended for specific purposes. These systems will also be subject to the requirements for ʻhigh-riskʼ AI systems if they can be used for ʻhigh-riskʼ applications. The specific details of these regulations are still being negotiated on a political level. However, it seems that complying with at least some key requirements of the AIA will likely be mandatory, unless the provider of a general-purpose AI system explicitly excludes all ʻhigh-riskʼ uses in the systemʼs instructions.
The second option is more cumbersome to explain, as it consists in a connection between the risk levels in the AIA and other NLF acts. The most significant NLF act in the present context is the MDR. In a slightly simplified manner, we can say the AIA classifies certain medical devices – considered particularly risky – as ʻhigh-riskʼ. To determine whether a medical device is ʻhigh-riskʼ, the AIA relies on one specific criterion: whether the MDR stipulates that a device is subject to a conformity assessment procedure involving a third party. The significance of conformity assessments is further explained below. At this point, it suffices to state that the AIAʼs risk classification rules directly connect to the MDR risk classification rules. This makes the classification rules in the MDR the central object of analysis when exploring the risk classification scheme for AI medical devices. In order to determine whether a medical AI system is regulated by the requirements of the AIA, we need to assess its classification under the MDR. This implies that the AI system must be classified as a medical device (as further discussed in Section 5) and, in addition, reach the MDR-specific risk threshold where the AI system is deemed risky enough to warrant the involvement of a third party in the conformity assessment of the system (discussed in Section 6).
5. The Medical Device Regulation and AI as a Software Medical Device
For stakeholders in the health sector, the AIA is a new layer of product safety legislation which is added on top of the MDR. The MDR lays down requirements to which medical devices must conform before they can be placed on the EU market. The MDR, besides governing manufacturers, also imposes specific obligations on distributors, importers and users, which include healthcare institutions. However, the manufacturer remains the most salient duty-subject under the MDR. Above all, the manufacturer is responsible for ensuring that their devices conform to the applicable MDR requirements. It is the manufacturer who initially determines which risk category a medical device belongs to. Thus, it is important that manufacturers have proper methods for assessing the risk posed by their devices.
AI medical devices, as defined in Section 3, are a subcategory of software medical devices and regulated as such under the MDR. A ʻmedical deviceʼ, according to Article 2(1) MDR, is ʻany instrument, apparatus, appliance, software […] intended by the manufacturer to be used, alone or in combination, for human beingsʼ for one or more specific medical purposes, including ʻdiagnosis, prevention, monitoring, prediction, prognosis, treatment or alleviation of diseaseʼ. Medical devices can, according to this definition, be as basic as hospital beds or face masks. Software systems are medical devices when they are intended to be used for medical purposes, unless their intended use is too generic; guidance from the Medical Device Coordination Group (MDCG) indicates that software systems only qualify as medical devices if they are intended for medical uses directed at individual patients. Thus, AI systems intended to be used to analyse large amounts of patient data to discover patterns and extract new knowledge, or to aid in strictly administrative decision-making processes, are not medical devices. For example, AI can be used to support decisions concerning high-level management of (human or other) resources, or to provide the basis for changes in how a health institution is organised or how health services are provided (eg, due to indications of a potential for quality improvement, increased efficiency, etc). Such AI systems can all be referred to as ʻmedical AIʼ, but they are not ʻmedical devicesʼ pursuant to the MDR. As a consequence, they would only be subject to the requirements for ʻhigh-riskʼ AI systems under the AIA if integrated in the list included in Annex III to the AIA.
An AI medical device may take the shape of an application that anyone can download and use anytime, anywhere, on their personal device (ʻmHealthʼ). An AI medical device may also be a software system operating on a computer in a physicianʼs office, in an intensive care unit or in another setting where a health institution and/or clinician is in charge of how the device is used. As long as an AI system that falls under the definition of a ʻmedical deviceʼ operates on a hardware device that is not in itself a medical device, the AI system constitutes a so-called ʻstandaloneʼ software medical device. For risk classification purposes, standalone software medical devices are classified in their own right under the MDRʼs risk classification scheme.
AI medical devices set themselves apart from conventional, hard-coded medical software systems. This is due to their capacity to learn from examples and formulate their own decision rules, rather than relying on human software engineers to generate decision rules for every potential scenario. Consequently, medical AI systems may present risks that differ from those posed by traditional software systems. The AIAʼs explanatory memorandum highlights several key traits of AI systems that are closely linked to safety and fundamental rights risks. These relate to ʻopacity, complexity, bias, a certain degree of unpredictability and partially autonomous behaviourʼ. Such traits may be especially pertinent to generative AI systems. Thus, to align with the objectives of the EU legislature in mitigating AI-specific risks, it is vital that the risk classification scheme appropriately addresses generative AI systems. Bearing this consideration in mind, we now turn our attention to the AIAʼs risk classification scheme for medical AI systems.
6. Risk Classification of Medical AI Systems
6.1 Introduction
As explained above, the AIA creates a risk classification scheme for medical AI which is intertwined with the MDR risk classification scheme. To assess how regulatory lacunae may exist in this intertwined scheme, it is necessary to understand the role played by the risk classification schemes of the AIA and MDR, respectively. Section 6.2 therefore describes the main features of the two risk classification schemes. On this basis, Section 6.3 addresses how the two frameworks are intertwined. A medical AI system potentially needs to be assessed under both the AIA and the MDR. A medical AI systemʼs classification as ʻhigh-riskʼ (thus triggering the AIAʼs requirements) depends on the risk class under the MDR. Therefore, it is only possible to verify the applicability of the AIA to medical AI systems, if both risk classification schemes are assessed in combination.
6.2 The Functions of Risk Classification Under the AIA and the MDR
Except for the fact that the AIA includes fundamental rights considerations, the concept of ʻriskʼ is similarly defined for the purposes of the MDR and the AIA. ʻRiskʼ is defined in the MDR as the ʻcombination of the probability of occurrence of harm and the severity of that harmʼ. This definition builds on a methodological approach to risk which was also used to define the ʻhigh-riskʼ category in the AIA. The safety and performance requirements that medical devices must conform to are intended to reduce risk by reducing the probability and impact of incidents that may have a negative impact on health and safety. ʻSafetyʼ in the MDR is closely connected with the performance of medical devices. ʻPerformanceʼ is defined as ʻthe ability of a device to achieve its intended purpose as stated by the manufacturerʼ. In a medical setting, when a device fails to fulfil the purpose it was designed for, the failure can easily pose a health and/or safety risk to persons who rely on the device. For example, lead fracture is a foreseeable, undesirable incident in pacemakers. If lead fracture occurs in a pacemaker, the electric impulses meant to stimulate the patientʼs heartbeats may not reach the heart chamber. The pacemaker will in this case not achieve its intended purpose of controlling the patientʼs heart rate, and the patient will be at increased risk of heart failure and death. The safety and performance requirements in the MDR are designed to mitigate the probability and severity of incidents like these, which are foreseen by the legislator. Similarly, the AIA is designed to minimize the probability and impact of such incidents during the use of AI systems. However, the AIA primarily aims at the undesirable incidents that are not already anticipated by existing product safety legislation.
Compared to the AIAʼs main dichotomy between ʻhigh-riskʼ and other systems, the MDRʼs risk classes are structured differently and perhaps slightly more elaborately. A medical device regulated under the MDR is assigned to one of four risk classes: class I, class IIa, class IIb, or class III. The classification rules are based on the vulnerability of the human body and take into account the potential risks associated with the technical design and manufacture of the devices. Accordingly, pacemakers and pacemaker leads are placed in the MDRʼs highest risk category (ref. the example just mentioned above). As a general rule (unless anything else is specified), medical devices are subject to the same substantive MDR requirements regardless of their risk classification. The manufacturer must ensure that medical devices in all risk classes conform to the general safety and performance requirements set out in Annex I MDR. The risk classification does not alter this responsibility. Thus, the function of the MDR risk classification is to determine which conformity assessment procedure the manufacturer must follow. As already hinted at, an especially important variable that changes depending on MDR risk classification of a medical device is the involvement of ʻnotified bodiesʼ in the conformity assessment procedure (further explained in Section 6.3).
The AIA and the MDR are both meant to strike a proportionate balance between the need to protect persons from the potential harms of the regulated products and the legislative aim of enhancing innovation and trade. In both frameworks, risk classification is the central tool to ensure proportionality. In addition to risk classification rules, the provisions that determine the scope of application of a product safety act can also contribute to ensuring proportionality. Arguably, the provisions that determine the scope of the AIA and the MDR, ie the definitions of ʻartificial intelligenceʼ and ʻmedical deviceʼ, play different roles when it comes to ensuring proportionality. The AIA has a wide scope of application, determined by the broad definition of ʻartificial intelligenceʼ. Given its wide scope of application, the AIAʼs risk classification scheme serves the task of ensuring proportionality by distinguishing between AI systems that should be subject to the material requirements in Title III and those that should not. In comparison, the MDRʼs definition of a ʻmedical deviceʼ is narrower and therefore plays a more important role in contributing to the proportionality of the MDR.
6.3 Medical AI as ʻHigh-Riskʼ AI Systems
We shall now turn to the details of how the AIA and the MDR are intertwined. The two acts are aligned through the definition of ʻhigh-riskʼ AI systems in the AIA, Article 6 (1). In brief, an AI system that is also a medical device, or a safety component thereof, only counts as ʻhigh-riskʼ (under the AIA) if the medical device is required to undergo a third-party conformity assessment under the MDR. This means that we need to assess the MDRʼs risk classification criteria in detail, focussing on medical AI systems.
As mentioned above, this article searches for lacunae that exist in the regulatory space between the AIA and the MDR. If a medical AI system does not count as a medical device at all, or if it falls outside the ʻhigh-riskʼ class in the AIA, then the requirements in the AIA do not apply to a medical AI system. Before diving into the MDRʼs risk classification rules in more detail, it must be reiterated that not all medical AI systems are necessarily medical devices. If a medical AI system is not a medical device, it must be on the list in Annex III of the AIA to be classified as ʻhigh-riskʼ. Because there are probably very few medical AI systems covered by the list of ʻhigh-riskʼ AI systems in Annex III, the most relevant criterion is the connection between the AIA and the MDR (and other similar acts) incorporated in Article 6 (1) of the AIA. Thus, the most practical criterion which determines whether a medical AI system shall be classified as ʻhigh-riskʼ under the AIA will in most cases be whether a third party is involved in the applicable MDR conformity assessment procedure. As further elaborated below, this depends on the risk classification under the MDR.
Under the MDR, the relevant third party would be a so-called ʻnotified bodyʼ. Notified bodies play an important role in the MDRʼs conformity assessment procedures. Their involvement depends on the type of device and its risk classification. Notified bodies are often, but not necessarily, private entities that are authorised by a Member Stateʼs competent authority to assess whether a medical device complies with the safety and performance requirements, mainly by reviewing the documentation provided by the manufacturer. To determine whether involvement of a notified body is required, one must consult the different conformity assessment procedures described in the annexes to the MDR. As a general rule, manufacturers are entitled to self-assess devices in class I (with a few exceptions that are not relevant to this article), whereas devices in higher risk classes are subject to conformity assessment procedures which entail the involvement of a notified body. Thus, notified bodies are always involved in the assessment of devices in class IIa, class IIb, or class III.
For AI medical devices, the general rule that notified bodies are not involved in the conformity assessment of devices in class I means that these AI medical devices are non-ʻhigh-riskʼ AI systems under the AIA. In contrast, AI medical devices in MDR class IIa, IIb or III are ʻhigh-riskʼ AI systems under the AIA and their providers must comply with the requirements laid down in Title III of the AIA. It is therefore pertinent to explore the rules that determine whether a medical device shall be placed in class I or higher according to the MDR. Once it is determined whether a device should be placed in MDR class I or in a higher class, it is not important for the purposes of this article which one of the higher classes the device belongs in. The reason for this is that once a medical AI system is covered by the MDR and placed in a higher class than class I, it can be presumed that the AI system is subject to material and procedural requirements which address traditional medical device risks (ie, the MDR requirements) as well as AI-specific risks posed by the system (the requirements for ʻhigh-riskʼ AI systems in the AIA). In other words, such an AI system is classified in accordance with the AIAʼs legislative intentions and does not expose the type of regulatory lacunae we are searching for in this article. We expect that most medical AI systems that pose significant safety risks will fall into the ʻhigh-riskʼ category. Searching for regulatory lacunae therefore means searching for the exceptions. With this in mind, we turn towards the classification rules in the MDR, which are set out in Annex VIII MDR. Medical devices that are not assigned to a higher risk class according to a specific classification rule belong in class I, according to the MDR. We therefore consider the relevant classification rules that might place AI medical devices in class IIa, class IIb, or class III. This is best explained in the specific context of case studies.
6.4 Introduction to Case Studies
To concentrate the search for regulatory lacunae around the risk classification of medical AI systems, this article considers the examples of therapeutic chatbots and automated documentation in electronic health records. These two examples are selected as case studies because they pose different types of health and safety risks which illustrate different aspects of the risk classification scheme, particularly in respect of medical AI systems with different levels of generativity. The following sections introduce the two case studies and the health and safety risks associated with them. As elaborated below, therapeutic chatbots present AI-specific risks, whereas EHR documentation systems present a more traditional type of risk associated with medical devices. We find upon a closer examination that the risks illustrated by the case studies indeed point towards potential regulatory lacunae at the intersection between the AIA and the MDR.
7. Case Study 1: Therapeutic Chatbots
7.1 The Case – Doctor Chatbot
By ʻtherapeutic chatbotsʼ we refer to conversational agents for automated, digitally provided therapy. Such conversational agents may take the shape of interactive applications based on text, audio, video or virtual reality. Research suggests that it is feasible to use various forms of chatbots or virtual avatars in the treatment of patients with conditions such as major depressive disorder, anxiety, schizophrenia, post-traumatic stress disorder and autism spectrum disorder. Therapeutic chatbots with a high degree of generativity can have fully automated interactions with individuals and mimic the style of therapeutic conversations that patients have with clinicians. They can be accessible to anyone through online marketplaces and function as self-management tools, in which case their status as medical devices may be ambiguous. They are nonetheless medical devices if they are intended for the treatment, prevention or monitoring of a medical condition. Therefore, providers of chatbots that are available to consumers often claim that the applications are intended to be used for informational purposes only, or for non-medical wellness purposes. For example, the intended purpose of AI-based chatbots for weight loss can easily be articulated along these lines to make sure the applications stay clear of the MDR. Similarly, ChatGPT serves a general purpose and does not fall under the definition of a medical device. In contrast, if the intention is that a therapeutic chatbot should be used as part of professional medical treatment, the chatbot is clearly a medical device under the MDR. Because chatbots run on non-medical hardware such as general-purpose computers, mobile devices, gaming consoles, virtual reality goggles, etc, they are regulated as standalone software devices under the MDR.
Joseph Weizenbaum, the creator of one of the earliest (rule-based) mental health chatbots (ELIZA), remarked that ʻextremely short exposures to a relatively simple computer program could induce powerful delusional thinking in quite normal peopleʼ. More recent literature on mental health chatbots points out particular risks related to AI-driven systems, suggesting that it can be challenging to ensure that AI-based chatbots provide adequate responses and to control the responses they provide. This insight mirrors one of the concerns raised in the AIAʼs Explanatory Memorandum, where AI systemsʼ partial autonomy is put forward as a source of risk. Another potential health and safety risk is related to the possibility that chatbot users may become dependent on access to chatbots, which can lead to increased addictive behaviour and avoidance of interaction with human clinicians. Moreover, several works in the field suggest that a potential for harm to patients arises due to chatbotsʼ limited ability to identify and respond to important indications such as mood changes, or even emergency situations, eg, where the patient is suicidal. It can even be envisaged that an AI-based chatbot that misinterprets a personʼs mental state during virtual therapy may provide responses that reinforce the deterioration, which can lead to fatal outcomes. These risks relate particularly to the generative nature of chatbots. Because the content of the therapy is generated autonomously in response to the information a chatbot draws from an individual, a chatbot may be unpredictable while also appearing to be convincing and dependable.
We will now assess the possible regulatory lacuna regarding therapeutic chatbots. The risks identified above provide an indication that there is some need for regulating these AI systems. The following discussion refers to therapeutic chatbots which constitute AI medical devices – they are covered by the MDRʼs definition of a ʻmedical deviceʼ. However, the AIA will only be applicable to therapeutic chatbots as ʻhigh-riskʼ AI systems, if they are placed in class IIa or higher under the MDR. Because the default Class I results in the AIA not being applicable to medical AI, we need to identify classification rules that could point to a higher class. Among the classification rules in Annex VIII MDR, Rule 11 and Rule 22 stand out as the relevant classification rules for AI medical devices.
7.2 MDR Rule 11: Software Medical Devices
MDR Rule 11 was designed to take into account the particularities of the risks presented by standalone software devices. The software-specific risks captured by Rule 11 are risks related to the consequences of the failure to provide correct information, when a software device is used to support clinical decisions. This type of risk differs from the risk associated with devices that are in closer contact with the patientʼs body and was included in the MDR because the legislator anticipated the increased use of software medical devices.
Rule 11 addresses two distinct categories of software medical devices: those that are commonly known as Decision Support Systems (DSS) and those that are known as Patient Monitoring Systems (PMS). More precisely, the DSS category addressed by Rule 11 is defined in the first paragraph as ʻSoftware intended to provide information which is used to take decisions with diagnosis or therapeutic purposesʼ. The PMS category is defined in the second paragraph as ʻSoftware intended to monitor physiological processesʼ. Medical device software not intended to be used for decision-making and/or monitoring purposes, are not specifically addressed by Rule 11, so they end up in the fall-back position of Class I. This is a result of the traditional assumption that the principal risk posed by standalone medical device software is related to the provision of erroneous information as a basis for decisions.
Decision support systems and patient monitoring systems are directly addressed by Rule 11 MDR, and will normally fall into class IIa or higher and, thus, be deemed as ʻhigh-riskʼ systems under the AIA. The problem is, however, that therapeutic chatbots do not appear to fall within the types of software devices that are assigned to higher risk categories according to Rule 11 MDR. They are therapeutic devices intended for the treatment or alleviation of mental health conditions. As such they enable a type of automated therapy that can be conducted without immediate oversight from a (human) clinician. They are not intended to be used primarily for decision support or patient monitoring purposes, so Rule 11 does not lead to a higher classification. Unless other classification rules lead to a different outcome, therapeutic chatbots end up in class I, which does not trigger the applicability of the AIAʼs requirements for ʻhigh-riskʼ AI systems. Because Rule 11 does not, in our view, place therapeutic chatbots in a higher risk class than class I, it is worth discussing whether therapeutic chatbots may be assigned to a higher risk class according to other parts of the MDR.
7.3 MDR Rule 22: Active Therapeutic Devices
In addition to Rule 11, which is specifically intended for software medical devices, Rule 22 may also be relevant to software devices. This rule places ʻactive therapeutic devices with an integrated or incorporated diagnostic function which significantly determines the patient management by the deviceʼ in Class III. Software devices are ʻactive devicesʼ. However, to be an ʻactive therapeutic deviceʼ, a device must be intended to ʻsupport, modify, replace or restore biological functions or structures with a view to treatment or alleviation of an illness, injury or disabilityʼ (our italicisation). Rule 22 could be challenging to apply, if therapeutic chatbots are primarily seen as focusing on mental, rather than biological functions.
It seems, nevertheless, pertinent to consider the application of Rule 22 to certain cognitive therapy devices in light of influential guidance presented by the Medical Device Coordination Group (MDCG). In MDCG-2019-11 (Guidance on Qualification and Classification of Software), the MDCG gives the following example:
Cognitive therapy MDSW that includes a diagnostic function which is intended to feed back to the software to determine follow-up therapy, e.g., software adapts treatment of depression based on diagnostic feedback.
According to the guidance, such cognitive therapy devices ʻshould be in class III per Rule 22ʼ. The example seems to refer to a type of device that might include AI-based therapeutic chatbots. It is possible that a chatbot or other software could modify biological functions, for example by automatically altering the dosage of specific medications. If this is the case, the guidance suggests that the software is classified in class III according to Rule 22. However, unless there is a clear impact of the therapy device on biological functions, it is unclear how Rule 22 can be applicable. The way we envisage therapeutic chatbots, they could continuously assess the userʼs mental state and autonomously adapt the therapy in accordance with its autonomous assessments. If the treatment provided by Doctor Chatbot is a form of cognitive behavioural therapy, for example, it does not seem fitting to state that the treatment modifies biological functions. Therefore, the Doctor Chatbot scenario does not fit well with the wording of Rule 22. Rule 22 only applies to ʻactive therapeutic devicesʼ, ie, devices intended to ʻsupport, modify, replace or restore biological functions or structures …ʼ. Despite this wording, the example in MDCG-2019-11, referred to above, suggests that Rule 22 may cover chatbots that do not affect biological functions or structures.
Regardless of exactly which devices the MDCG referred to with the abovementioned example, our point is that the application of Rule 22 to therapeutic chatbots seems challenging and unclear. Moreover, the automated cognitive therapy example from the 2019 guidance is not mentioned in the MDCGʼs most recent guidance on classification of medical devices (MDCG 2021-24). In the 2021 guidance, the MDCG refers to examples that lie more firmly within a reasonable understanding of ʻbiological functionsʼ (inter alia; automated external defibrillators, automated closed-loop insulin delivery systems, and closed-loop systems for deep brain stimulation). It is, therefore, not clear whether the MDCG maintains the view that Rule 22 applies to cognitive therapy devices, including therapeutic chatbots.
Based on a reasonable distinction between biological and cognitive functions, we find that Rule 22 cannot be applied to cognitive therapy devices such as therapeutic chatbots. We therefore must conclude that therapeutic chatbots are not assigned to higher risk classes by Rule 22. Nor are they subject to other MDR classification rules, as they do not pose the types of risk accounted for by those rules (eg, radiation emission, toxic substances or physical invasiveness). Accordingly, they must be placed in MDR class I. Consequently, they would not be subject to the requirements that apply to ʻhigh-riskʼ AI systems pursuant to the proposed AIA.
7.4 Conclusion for Therapeutic Chatbots
The concerns that have been flagged in the chatbot literature (reviewed at the outset of Section 7) indicate that there are ways in which therapeutic chatbots could fail and cause harm to patientsʼ health and safety. From a policy standpoint it is, therefore, understandable that the MDCG recommended in its 2019 guidance that cognitive therapy devices should be placed in MDR class III. As argued in the previous section, however, the classification rule for automated therapeutic devices (Rule 22) anticipates only the harms associated with impacts on biological functions, and Rule 11 anticipates harms related to the provision of erroneous information as the basis for decision-making. The risks of automated cognitive therapy seem to elude these anticipations of the legislative risk assessment which underpins the MDR. In theory, because the risks posed by therapeutic chatbots are largely related to characteristics that are typical for AI systems – autonomy, unpredictability, and lack of human oversight – it lies within the purpose of the AIA to capture and counteract these risks. Yet, in practice, by giving complete deference to the MDRʼs risk classification scheme, the AIA is not applicable and thus does not close this regulatory lacuna.
8. Case study 2: An Automated Health Record Documentation System
8.1 The Case
In digitalised health systems, the electronic health record (EHR) is an important source of information that clinicians rely on for their treatment of patients. It is therefore important that the EHR contains updated and correct information. To ensure that the EHR contains the relevant information, clinicians must in practice manually register the information collected during each encounter with a patient. The process of registering patient information in the EHR is known to be time-consuming for clinicians, taking up a significant portion of their workday. In practice, clinicians register some information in EHR systems during the interaction with a patient and some information between seeing different patients. Inevitably, the information that clinicians can register will be limited by time constraints and their ability to recall the conversation with the patient. To reduce time spent typing information into the EHR and to enhance the completeness of the registered information, an AI system for automated documentation may be applied. Using Natural Language Processing, an AI-based speech recognition system can listen to the patient consultation and automatically input structured (according to pre-defined tables, etc) and unstructured (free text) information into the EHR. In its simplest form, such a system could simply transcribe spoken words into text to be archived. In a more advanced version, we can envisage that these inputs might be used as prompts for a specialised generative AI that is capable of generating relevant text, such as summaries and cliniciansʼ assessments, to be entered into the EHR.
Safety concerns have already been raised in relation to automated EHR documentation due to the potential harms that patients may incur as a result of errors in these systems. Among other risks, whenever an EHR contains incorrect or incomplete information, there is a potential for mistakes and suboptimal treatment down the line. Medical personnel relying on the EHR may reach other decisions than they would have done if the information had been correct. This is a type of error and risk that occurs also with traditional EHR documentation methods. This case study is therefore illustrative of a type of risk that may be posed by an AI system, but which is not specific to AI systems. In fact, the type of risk posed by an automated EHR documentation system is addressed by Rule 11 MDR, which refers to software ʻintended to provide information which is used to take decisions with diagnosis or therapeutic purposesʼ. A central purpose of EHR documentation is to provide the informational foundation for clinical decisions. If such a system qualifies as a medical device, it would therefore be placed in a higher risk class than Class I according to Rule 11, and consequently, it would be a ʻhigh-riskʼ AI system under the AIA.
8.2 Risk Classification of an Automated EHR Documentation System
As illustrated by the therapeutic chatbots example, AI medical devices escape the AIAʼs ʻhigh-riskʼ category if they belong in MDR class I. This will be the case if an AI medical device poses risks that are not foreseen by the MDRʼs risk classification rules. Another path around the AIAʼs ʻhigh-riskʼ category is for an AI system to stay clear of the MDR altogether, by not being defined as a medical device.
If a medical AI system is not a ʻmedical deviceʼ, it can only be a ʻhigh-riskʼ AI system if it is covered by the list of ʻhigh-riskʼ systems in the AIAʼs Annex III. Given our search for regulatory lacunae, the question is whether there may be medical AI systems that pose significant health and safety risks, that are not medical devices. Section 8.1 introduced the prospect of an AI system for automated documentation in EHRs and established indications of considerable health risks associated with the malfunctioning of such a system. The risks are related to the provision of erroneous information as a basis for decision-making, which is the main software-related risk anticipated by the MDR (Rule 11). However, if the AI system is not a medical device, it will still fall outside of the AIAʼs ʻhigh-riskʼ category (see Section 6). Thus, when the AIAʼs risk classification scheme is applied to the case of AI-based EHR documentation, the classification as ʻhigh-riskʼ hinges just as much on the definition of a ʻmedical deviceʼ as it does on the MDRʼs classification rules. If the system is not a ʻmedical deviceʼ according to Article 2(1) MDR, it is not classified as a ʻhigh-riskʼ AI system according to the proposed AIA.
In SNITEM, the CJEU indicated that a software is not a ʻmedical deviceʼ if it ʻhas the sole purpose of archiving, collecting and transmitting data, like patient medical data storage software…ʼ. It further suggested that software can, without becoming a ʻmedical deviceʼ, be intended to perform actions on data in a medical context if those actions are ʻlimited to storage, archiving, lossless compression or, finally, simple search […] without modifying or interpreting itʼ. It is not clear what this indicates for generative AI systems designed to automatically collect and insert information into the EHR. On the one hand, the system would primarily be meant to archive, collect, and transmit data. On the other hand, any translation of human speech to data and text can be said to involve an element of interpretation and modification. Arguably, the directions given by the CJEU in SNITEM would suggest that the degree of generativity in an AI system is a decisive factor that may determine whether the AI system is a medical device. It is therefore important to consider the extent to which an AI system is intended to engage with the data that it processes. In practice, different levels of generativity are conceivable, ranging from simple transcription, through translation, to more advanced systems capable of not only modifying content in various ways but also generating new content.
8.3 Conclusion for Case Study 2
The degree of generativity in an AI system for automated EHR documentation can determine whether the system qualifies as a medical device under the MDR and whether the main requirements in the AIA apply to the system. Arguably, the directions drawn up by the CJEU for the assessment of whether a software is a medical device indicate a low threshold of generativity; if a software systemʼs data processing involves only a modicum of interpretation or modification, it seems likely that the system should be deemed as a medical device. However, erroneous information outputted from an AI system can have serious consequences regardless of the systemʼs degree of generativity. From a safety perspective, the transcription of spoken language into text (low generativity) poses risks that are similar to those posed by more generative systems. This arguably represents an example of a borderline case where an AI system with health and safety impacts may or may not qualify as a medical device. Other borderline cases may occur as AI systems continue to spread throughout the health sector.
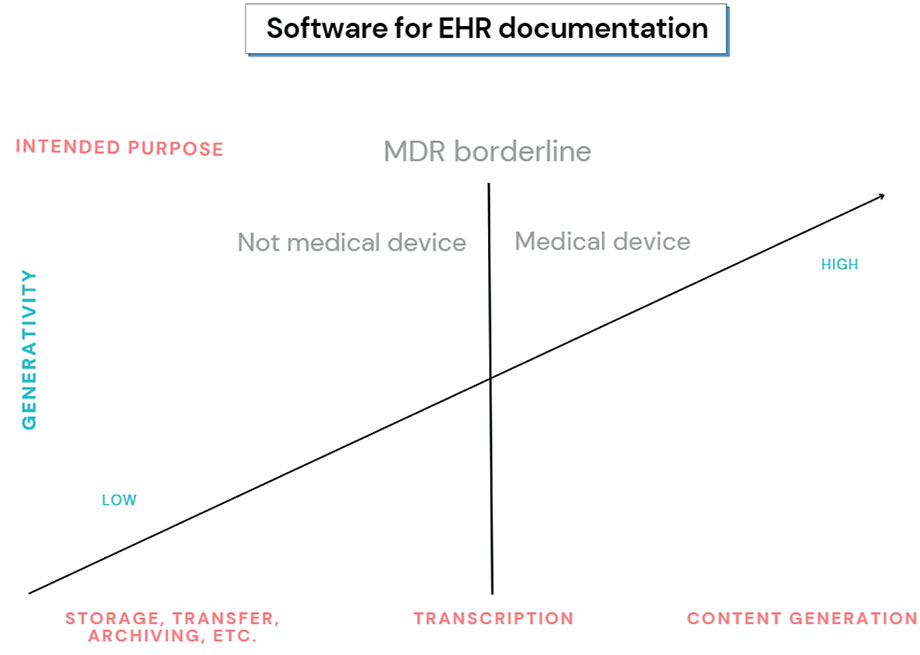
Figure 1
The figure illustrates how the degree of generativity can determine whether a software for EHR documentation constitutes a medical device according to the MDR.
9. Discussion
The EU legislatureʼs anticipation of product risks is crucial to facilitate a marketplace where citizens can trust that the available products are safe to use. AI products may present new risks that are specific to AI technologies and risks that are similar to well-known risks associated with existing product types. The proposed AIA is meant to protect EU citizens from AI-specific risks. When it comes to more traditional risks, the AIA relies on existing EU product safety legislation (the NLF).
There are good reasons why the AIA relies on existing product safety legislation. When an AI system is regulated by an existing NLF act, it means that the AI system falls into a category of products generally associated with certain safety risks. Those safety risks are addressed by the existing requirements and conformity assessment procedures for the relevant product category. A significant infrastructure, involving public authorities and private industry, is already established around the process of verifying different productsʼ conformity with the applicable requirements. Therefore, it is perfectly reasonable for the EU legislature to focus its legislative and regulatory efforts on new, AI-specific risks. However, this article has demonstrated how the AIAʼs risk classification scheme for medical devices may potentially fail to capture certain AI-specific risks as well as certain traditional risks associated with medical devices. In other words, we have identified potential regulatory lacunae that are not filled in by the proposed AIA.
First, this article has demonstrated that certain AI medical devices that pose AI-specific risks might not be subject to the AIA requirements, even though those requirements are designed to mitigate such risks. This is because the MDR risk classification is decisive for their applicability. The MDRʼs risk classification scheme primarily focuses on risks arising from inaccurate information used as a basis for clinical decisions. However, some AI medical devices may present AI-specific risks unrelated to the provision of inaccurate information and could be classified as class I under the MDR. These risks may not be captured by the risk classification scheme for AI medical devices, as a result of the AIAʼs reliance on the MDR classification scheme. The implications of this issue have been illustrated by the example of AI-based therapeutic chatbots. The potential for AI-based therapeutic chatbots operating with limited clinical oversight has been fortified by recent advancements in generative LLMs and is not anticipated by the MDR.
Second, the article has confirmed that the risk classification scheme for medical AI systems opens up to a potential regulatory lacuna at the outskirts of the MDRʼs area of application: medical AI systems not mentioned in Annex III of the AIA are only placed in the AIAʼs ʻhigh-riskʼ category if they are defined as medical devices. Therefore, medical AI systems that are not medical devices escape the requirements for ʻhigh-riskʼ AI systems, even if they pose more traditional types of risks, ie risks that the MDR is designed to mitigate. This leaves the AIAʼs risk classification scheme vulnerable to speculative formulations of a medical AI systemʼs intended purpose. While others have made this point in relation to potentially risky wellness applications, which may be marketed as being intended for non-medical purposes, we have shown that the intended generativity of a medical AI system may determine whether it constitutes a medical device. Different levels of generativity can produce borderline cases that may be challenging to regulate. In borderline cases, providers might be inclined to downplay the degree of generativity in a medical AI system, thus evading MDR and AIA requirements.
To address the gap concerning medical AI systems falling outside the scope of the MDR, one specific solution proposed in the literature is to add these systems to the list of ʻhigh-riskʼ AI systems in Annex III of the AIA. This approach suggests including ʻmedical or health-related AIʼ in the list, which would effectively address the challenges outlined in this article. However, it is important to evaluate carefully whether this solution may generate new challenges. If all health-related AI systems are categorically defined as ʻhigh-riskʼ, there would be no opt-out for providers of applications that operate at the periphery of the medical context and potentially pose lower risks compared to other systems listed in Annex III. Such a broad inclusion would undoubtedly create compliance challenges for a diverse and loosely defined set of AI systems connected to the health sector. Therefore, justification for this inclusion would be warranted only if these systems exhibit a high level of risk specifically associated with their utilisation of AI.
10. Conclusion and Recommendation
The EU legislature and national legislators are advised to consider the possibility of medical AI systems that either fall into MDR class I or fall outside the scope of the MDR because they are not medical devices. Because the AIA relies on MDR classification, these systems are not covered by the AIAʼs ʻhigh-riskʼ category, unless they are listed in Annex III of the AIA. To improve the risk classification scheme for medical AI in terms of capturing relevant health and safety risks, there are at least two approaches that may be considered: (i) the addition of certain (but not necessarily all) medical AI systems to the list of ʻhigh-riskʼ AI systems in Annex III of the AIA; and (ii) the addition of AI-specific classification rules in the MDR (at least to the extent needed to address therapeutic chatbots).
In relation to option (i), it was noted in section 9 above that an inclusion of all medical AI systems in the AIAʼs list of ʻhigh-riskʼ systems might not be justified. An alternative solution could be to use the MDRʼs definition of a medical device as a foundation for a more targeted and specific inclusion of essential medical purposes within Annex III of the AIA. This solution would imply that all AI medical devices, but not all medical AI systems, should be placed in the AIAʼs ʻhigh-riskʼ category. A key advantage of this solution is that it enables the AIAʼs risk classification scheme to capture a medical AI systemʼs degree of generativity (which is associated with AI-specific risk). In our interpretation of a ʻmedical deviceʼ under the MDR, we found that the degree of generativity may be a decisive criterion.
If the EU legislature prefers for the AIA to give deference to the MDRʼs risk classification scheme, rather than adding certain medical AI systems to the list in Annex III of the AIA, option (ii) may be chosen. To address the problem of AI medical devices potentially being classified as low risk (MDR class I), the MDRʼs classification rules could be amended with a risk classification rule for generative AI medical devices capable of providing treatment responses autonomously. This would be an even more targeted solution than option (i), with less risk of unintentionally subjecting low-risk AI systems to ʻhigh-riskʼ requirements. However, this approach would not remedy the lacunae of risky medical AI systems that are potentially not defined as medical devices. To some extent, it may be possible to mend this gap through teleological interpretation of the MDRʼs definition of a ʻmedical deviceʼ. Given the MDRʼs objective to effectively manage specific medical risks, a teleological interpretation could permit those risks to be considered when assessing whether an AI system is a medical device. For example, it could be argued that the borderline case of a low-generativity AI system for EHR documentation should be deemed a medical device because it poses a type of risk that is typical of medical devices (the risk of erroneous information serving as a basis for clinical decisions). By incorporating risk considerations into the interpretation of the ʻmedical deviceʼ definition, the MDR could uphold proportionality between innovation and regulation, while remaining aligned with its intended objectives.
Funding and Acknowledgments
Parts of this research are supported by the project ʻData-Driven Health Technologyʼ (project number 310230101), funded by UiT the Arctic University of Norway, and the VIROS project, funded by the Norwegian Research Council (project number 247947). We thank Timo Minssen for extensive comments and suggestions to earlier versions of the manuscript. We also thank Christian Johner and Brita Elvevåg for valuable comments to an earlier version. Finally, we thank the reviewer for insightful comments. Any remaining errors are the sole responsibility of the authors.
- 1For example, the World Health Organization (WHO) discusses various issues in a guidance document: see World Health Organization, ʻEthics and governance of artificial intelligence for health: WHO guidanceʼ (2021). Also, van Kolfschooten outlines potential impacts on patient rights: see Hannah van Kolfschooten, ʻEU Regulation of Artificial Intelligence: Challenges for Patientsʼ Rightsʼ (2022) 59(1) Common Market Law Review 81 <https://doi.org/10.54648/cola2022005>.
- 2European Commission, Proposal for a Regulation of the European Parliament and of the Council Laying Down Harmonised Rules on Artificial Intelligence (Artificial Intelligence Act) and Amending Certain Union Legislative Acts (COM(2021) 206 final). At the time of this articleʼs publication, the AIA has not yet been adopted, but the trialogue process has begun. Unless otherwise noted, all subsequent references to the AIA pertain to this Commission Proposal.
- 3Regulation (EU) 2017/745 of the European Parliament and of the Council of 5 April 2017 on medical devices, amending Directive 2001/83/EC, Regulation (EC) No 178/2002 and Regulation (EC) No 1223/2009 and repealing Council Directives 90/385/EEC and 93/42/EEC [2017] OJ L 117/1.
- 4Sofia Palmieri and Tom Goffin, ʻA Blanket That Leaves the Feet Cold: Exploring the AIA Safety Framework for Medical AIʼ (2023) 30 European Journal of Health Law 1 <https://doi.org/10.1163/15718093-bja10104>. De Cooman also makes the point that there may be risky systems that fall outside of the AIAʼs ʻhigh-riskʼ category. His example is consumer recommender systems: see Jerome De Cooman, ʻHumpty Dumpty and High-Risk AI Systems: The Ratione Materiae Dimension of the Proposal for an EU Artificial Intelligence Actʼ (2022) 6(1) Market & Competition Law Review 49 <doi.org/10.34632/mclawreview.2022.11304>.
- 5ML is an advanced form of data analysis that is used to generate models through iterative exposure of a learning algorithm to data. The resulting model can be implemented in a software system that can provide a meaningful output when prompted with input information. See further eg Jenna Burrell, ʻHow the machine “thinks”: Understanding opacity in machine learning algorithmsʼ (2016) 3(1) Big Data & Society 1, 5ff <https://doi.org/10.1177/2053951715622512>.
- 6While often based on deep learning, a subset of machine learning, NLP is an umbrella term that covers many different computational techniques for analysis and representation of language: see Uday Kamath, John Liu and James Whitaker, Deep Learning for NLP and Speech Recognition (Springer 2019) 11-15.
- 7Ștefan Busnatu and others, ʻClinical Applications of Artificial Intelligence—An Updated Overviewʼ (2022) 11 Journal of Clinical Medicine 2265 <https://doi.org/10.3390/jcm11082265>; Thomas Davenport and Ravi Kalakota, ʻThe potential for artificial intelligence in healthcareʼ (2019) 6 Future Healthcare Journal 94 <https://doi.org/10.7861/futurehosp.6-2-94>.
- 8Joseph C Ahn and others, ʻThe application of artificial intelligence for the diagnosis and treatment of liver diseasesʼ (2021) 73 Hepatology 2546 <https://doi.org/10.7861/futurehosp.6-2-94>; Dong-Ju Choi and others, ʻArtificial intelligence for the diagnosis of heart failureʼ (2020) 3(54) npj Digital Medicine 1 < https://doi.org/10.1038/s41746-020-0261-3>; Jonathan T Megerian and others, ʻEvaluation of an artificial intelligence-based medical device for diagnosis of autism spectrum disorderʼ (2022) 5(1) npj Digital Medicine 57 <https://doi.org/10.1038/s41746-022-00598-6>.
- 9Alessandro Allegra and others, ʻMachine Learning and Deep Learning Applications in Multiple Myeloma Diagnosis, Prognosis, and Treatment Selectionʼ (2022) 14(3) Cancers 606 <https://doi.org/10.3390/cancers14030606>; Kipp W Johnson and others, ʻArtificial intelligence in cardiologyʼ (2018) 71(23) Journal of the American College of Cardiology 2668 <https://doi.org/10.1016/j.jacc.2018.03.521>.
- 10Riccardo Miotto and others, ʻDeep learning for healthcare: review, opportunities and challengesʼ (2018) 19(6) Briefings in bioinformatics 1236 <https://doi.org/10.1093/bib/bbx044>.
- 11Nenad Tomašev and others, ʻA clinically applicable approach to continuous prediction of future acute kidney injuryʼ (2019) 572(7767) Nature 116 <https://doi.org/10.1038/s41586-019-1390-1>; Karl Øyvind Mikalsen and others, ʻUsing anchors from free text in electronic health records to diagnose postoperative deliriumʼ (2017) 152 Computer Methods and Programs in Biomedicine 105 <https://doi.org/10.1016/j.cmpb.2017.09.014>.
- 12Melanie Reuter-Oppermann and Niklas Kühl, ʻArtificial intelligence for healthcare logistics: an overview and research agendaʼ in Malek Masmoudi, Bassem Jarboui and Patrick Siarry (eds), Artificial Intelligence and Data Mining in Healthcare (Springer 2021) 1 <https://doi.org/10.1007/978-3-030-45240-7_1>; Amy Nelson and others, ʻPredicting scheduled hospital attendance with artificial intelligenceʼ (2019) 2 npj Digit Med 26 <https://doi.org/10.1038/s41746-019-0103-3>.
- 13Busnatu and others (n 7).
- 14Miotto and others (n 10) 1241; Zineb Jeddi and Adam Bohr, ʻRemote patient monitoring using artificial intelligenceʼ in Adam Bohr and Kaveh Memarzadeh (eds), Artificial Intelligence in Healthcare (Elsevier 2020) 203.
- 15Christoph Lutz and Aurelia Tamò, ʻRobocode-ethicists: privacy-friendly robots, an ethical responsibility of engineers?ʼ (2015) Proceedings of the ACM Web Science Conference 1 <http://dx.doi.org/10.1145/2786451.2786465>.
- 16Catherine Diaz-Asper and others, ʻA framework for language technologies in behavioral research and clinical applications: Ethical challenges, implications and solutionsʼ, American Psychologist (forthcoming).
- 17Regulation (EU) 2016/679 of the European Parliament and of the Council of 27 April 2016 on the protection of natural persons with regard to the processing of personal data and on the free movement of such data, and repealing Directive 95/46/EC (General Data Protection Regulation) [2016] OJ L 119/1.
- 18On the role of risk assessments in the regulatory and legislative process, see Julia Black, ʻThe role of risk in regulatory processesʼ in Robert Baldwin, Martin Cave and Martin Lodge (eds), The Oxford handbook of regulation (Oxford University Press 2010) 302.
- 19Directive 2009/48/EC of the European Parliament and of the Council of 18 June 2009 on the Safety of Toys [2009] OJ L 170/1.
- 20MDCG 2019-11 Guidance on Qualification and Classification of Software in Regulation (EU) 2017/745 – MDR and Regulation (EU) 2017/746 – IVDR (2019) 17. The Medical Device Coordination Group (MDCG) is an advisory body providing non-binding guidance on the interpretation of the MDR, to facilitate harmonised implementation of the regulation.
- 21European Commission (n 2).
- 22The provider is a natural or legal person that has an AI system developed with a view to placing it on the market or putting it into service under its own name or trademark, cf Article 3(2) AIA. It follows from Article 24 AIA that manufacturers of products covered by NLF acts (including the MDR) are subject to the providerʼs obligations under the AIA.
- 23Decision No 768/2008/EC of the European Parliament and of the Council of 9 July 2008 on a common framework for the marketing of products, and repealing Council Decision 93/465/EEC [2008] OJ L 218/82.
- 24European Commission (n 2), see especially Preamble Recitals 1, 2, 5, 10, 13, 15 and 27.
- 25ibid Explanatory Memorandum 3 emphasises that the AIA shall not impose requirements that would make it unnecessarily difficult or costly to place AI systems on the common market.
- 26Ensuring that AI systems are safe and respect fundamental rights, and enhancing the effective enforcement of applicable safety requirements and existing law on fundamental rights, are among the key objectives of the AIA: ibid Explanatory Memorandum 3.
- 27In addition to the AIA, AI-specific risks will be addressed by the proposed General Product Safety Regulation (European Parliament Legislative Resolution of 30 March 2023). As stipulated in Article 7(1)(h), this Regulation mandates that safety assessments must also consider the evolving, learning, and predictive functionalities of a product.
- 28European Commission, Impact assessment accompanying the Proposal for a Regulation of the European Parliament and of the Council Laying Down Harmonised Rules on Artificial Intelligence (Artificial Intelligence Act) and Amending Certain Union Legislative Acts (21 April 2021).
- 29See Article 5 AIA. The list of prohibited practices is exhaustive, but the Commission may extend the list in accordance with further rules laid down in the AIA proposal. As noted by Mahler, the prohibited practices are not explicitly assigned to a risk category, but it can be inferred that they are seen as representing an unacceptable level of risk: Tobias Mahler, ʻBetween risk management and proportionality: The risk-based approach in the EUʼs Artificial Intelligence Act Proposalʼ in Liane Colonna and Stanley Greenstein (eds), Law in the Era of Artificial Intelligence (The Swedish Law and Informatics Research Institute 2022) 248 <https://doi.org/10.53292/208f5901.38a67238>.
- 30See also Palmieri and Goffin (n 4) 14–15.
- 31Article 6 AIA.
- 32For example, it might be relevant to examine whether some therapeutic chatbots could violate the prohibition contained in Article 5(1)(a) AIA, if the AI system ʻdeploys subliminal techniques beyond a personʼs consciousness in order to materially distort a personʼs behaviour in a manner that causes or is likely to cause that person or another person physical or psychological harmʼ.
- 33Article 52 AIA foresees transparency obligations for certain AI systems below the ʻhigh-riskʼ threshold. These could apply to therapeutic chatbots, but transparency about the fact that a human is communicating with an AI, and not a human being, offers only a limited contribution to the management of the risks of these systems. In addition, the EU Parliamentʼs proposed amendments to the AIA include, in Article 4a, a set of general principles applicable to all AI systems: European Parliament, Amendments adopted by the European Parliament on 14 June 2023 on the proposal for a regulation of the European Parliament and of the Council on laying down harmonised rules on artificial intelligence (Artificial Intelligence Act) and amending certain Union legislative acts (COM(2021)0206 – C9-0146/2021 – 2021/0106(COD)).
- 34Article 16 AIA.
- 35Articles 10–15 AIA.
- 36Article 16 AIA, cf Articles 19 and 43 AIA.
- 37Article 48 AIA.
- 38Also, the AIA introduces fundamental rights risks to the risk classification scheme. However, further discussion of this component lies outside the scope of this article.
- 39Article 6(2), cf Annex III AIA.
- 40It should be noted that some medical AI systems, for example in hospitals, might be used to manage ʻaccess to and enjoyment of essential private services and public services and benefitsʼ, which would fall into the ʻhigh-riskʼ category pursuant to Annex III AIA, Section 5 (n 2). In addition, the European Parliamentʼs proposed amendments (n 33) to Annex III AIA, Section 5 c, classify emergency healthcare patient triage systems as ʻhigh-riskʼ AI systems.
- 41See Article 6(1) AIA.
- 42Article 13 (general obligations of importers) and Article 14 (general obligations of distributors) MDR. Health institutions are subject to various requirements throughout the MDR.
- 43Pursuant to Article 2(30) MDR, a ʻmanufacturerʼ is a natural or legal person who manufactures or fully refurbishes a device or has a device designed, manufactured or fully refurbished, and markets that device under its name or trademark.
- 44Bernhard Lobmayr, ʻAn Assessment of the EU Approach to Medical Device Regulation against the Backdrop of the US Systemʼ (2010) 1 European Journal of Risk Regulation 137, 143 <https://doi.org/10.1017/S1867299X00000222>.
- 45Lukas Peter and others, ʻMedical Devices: Regulation, Risk Classification, and Open Innovationʼ (2020) 6(2) Journal of Open Innovation: Technology, Market, and Complexity 42 <https://doi.org/10.3390/joitmc6020042>.
- 46The MDCG defines software as a set of instructions that processes input data and creates output data: see MDCG (n 20), 5.
- 47Article 2(1) MDR. The definition also includes products intended for diagnosis, prevention, monitoring, treatment, alleviation or compensation in relation to injury or disability.
- 48MDCG (n 20) Section 3.3. The guidance is non-binding, but it is in line with the CJEUʼs case law under the former Medical Device Directive: see Case C-329/16 Syndicat national de lʼindustrie des technologies médicales (SNITEM) [2017] ECLI:EU:C:2017:947.
- 49Article 2(1) MDR; Timo Minssen, Marc Mimler and Vivian Mak, ʻWhen Does Stand-Alone Software Qualify as a Medical Device in the European Union?—The CJEUʼs Decision in Snitem and What it Implies for the Next Generation of Medical Devicesʼ (2020) 28 Medical Law Review 615 <https://doi.org/10.1093/medlaw/fwaa012>.
- 50mHealth can be defined as health practices ʻsupported by mobile devices, such as mobile phones, patient monitoring devices, personal digital assistants (PDAs), and other wireless devicesʼ: see WHO Global Observatory for eHealth, mHealth: new horizons for health through mobile technologies: second global survey on eHealth (WHO 2011) 6. For examples of standalone mHealth software devices, including Appleʼs ECG app, see Sara Gerke, ʻDigital Home Health During the COVID-19 Pandemic: Challenges to Safety, Liability, and Informed Consent, and the Way to Move Forwardʼ in Carmel Shachar and others (eds), The Future of Medical Device Regulation: Innovation and Protection (Cambridge University Press 2022) 145.
- 51For more specific examples of AI medical devices, see Stan Benjamens, Pranavsingh Dhunnoo and Bertalan Meskó, ʻThe state of artificial intelligence-based FDA-approved medical devices and algorithms: an online databaseʼ (2020) 3 npj Digit Med 118 3, 3-4 <https://doi.org/10.1038/s41746-020-00324-0>.
- 52Timo Minssen and others, ʻRegulatory responses to medical machine learningʼ (2020) 7 Journal of Law and the Biosciences 1, 5 <https://doi.org/10.1093/jlb/lsaa002>.
- 53Annex VIII MDR, Section 3.3 of Chapter II. In contrast, software systems that drive or influence the use of a device shall be classified in accordance with the device.
- 54Daniel Schönberger, ʻArtificial intelligence in healthcare: a critical analysis of the legal and ethical implicationsʼ (2019) 27 International Journal of Law and Information Technology 171 <https://doi.org/10.1093/ijlit/eaz004>; WHO (n 1).
- 55European Commission (n 2), Explanatory Memorandum 2.
- 56Article 2(22) MDR.
- 57Article 2(23) MDR.
- 58European Commission, Impact Assessment Annexes Accompanying the Proposal for a Regulation of the European Parliament and of the Council laying down harmonised rules on artificial intelligence (Artificial Intelligence Act) and amending certain union legislative acts (2021) Annex 5.
- 59Anastasiya Kiseleva, ʻAI as a Medical Device: Is It Enough to Ensure Performance Transparency and Accountability in Healthcare?ʼ (2020) 4(1) European Pharmaceutical Law Review 5, 13 <https://doi.org/10.21 552/epIr/2020/1/4>.
- 60Article 2(22) MDR.
- 61Muhammad Talha Liaquat and Talal Alzahrani, ʻPacemaker Malfunctionʼ (StatPearls Publishing 2020) <https://europepmc.org/article/nbk/nbk553149>.
- 62Preamble Recital 58 MDR.
- 63Rule 7 (temporary pacemaker leads) and Rule 8 (implantable cardiac pacemakers or leads) of Annex VIII MDR, cf Medical Device Coordination Group, MDCG 2021-24 Guidance on Classification of Medical Devices (2021) 36–38.
- 64The exact definition of the scope of the AIA is being negotiated at a political level at the time of writing. Although the EU Council has proposed a somewhat narrower definition compared to the initial proposal from the European Commission (n 2), the Councilʼs definition remains relatively broad: see Council of the European Union, Proposal for a Regulation of the European Parliament and of the Council laying down harmonised rules on artificial intelligence (Artificial Intelligence Act) and amending certain Union legislative acts – General approach (2022).
- 65As argued in Mahler (n 29) 265–267.
- 66Article 53 MDR. Software devices are active devices according to Article 2(4) MDR.
- 67Article 35 MDR; John Chai, ʻRegulation of Medical Devices in the European Unionʼ (2000) 21(4) Journal of Legal Medicine 537, 545 <https://doi.org/10.1080/019476400455799>.
- 68Preamble Recital 60 MDR. Classification of devices is regulated in Article 51 MDR, and shall be carried out in accordance with Annex VIII, which further delineates the classification rules.
- 69See Article 8 AIA.
- 70For software devices, this is specified in Rule 11, third paragraph, Annex VIII MDR.
- 71The feasibility of using such chatbots is pending further research. Some commentators have noted that their deployment may not happen in the immediate future: see Simon DʼAlfonso, ʻAI in mental healthʼ (2020) 36 Current Opinion in Psychology 112, 113 <https://doi.org/10.1016/j.copsyc.2020.04.005>. However, it is possible that more recent developments in generative AI and LLMs can speed up the pathway towards deployment of chatbots in clinical practice: see eg Heather Landi, ʻGoogle, Microsoft and startups test out generative AI in healthcareʼ (Fierce Healthcare, 14 April 2023) <www.fiercehealthcare.com/health-tech/google-microsoft-and-startups-test-out-generative-ai-healthcare> accessed 2 May 2023.
- 72Aditya Nrusimha Vaidyam and others, ʻChatbots and Conversational Agents in Mental Health: A Review of the Psychiatric Landscapeʼ (2019) 64 The Canadian Journal of Psychiatry 456, 458-459 <https://doi.org/10.1177/0706743719828977>.
- 73John Torous and others, ʻThe growing field of digital psychiatry: current evidence and the future of apps, social media, chatbots, and virtual realityʼ (2021) 20 World Psychiatry 318, 321 <https://doi.org/10.1002/wps.20883>.
- 74Eileen Bendig and others, ʻThe Next Generation: Chatbots in Clinical Psychology and Psychotherapy to Foster Mental Health – A Scoping Reviewʼ (2019) Verhaltenstherapie 64 <https://doi.org/10.1159/000501812>.
- 75Tools in this category are already available, and their status as medical devices may be uncertain: see James A Armontrout and others, ʻCurrent Regulation of Mobile Mental Health Applicationsʼ (2018) 46 Journal of the American Academy of Psychiatry and the Law Online 204 <https://doi.org/10.29158/jaapl.003748-18>.
- 76US Food & Drug Administration, ʻExamples of Mobile Apps That Are NOT Medical Devicesʼ (US Food & Drug Administration 2018) <https://www.fda.gov/medical-devices/device-software-functions-including-mobile-medical-applications/examples-mobile-apps-are-not-medical-devices>; Eugenio Mantovani and Pedro Cristobal Bocos, ʻAre mHealth apps safe? The intended purpose rule, its shortcomings and the regulatory options under the EU medical device frameworkʼ in Hannah R Marston, Shannon Freeman and Charles Musselwhite (eds), Mobile e-Health (Springer 2017) 251, 253.
- 77Article 2(1) MDR.
- 78See generally Mantovani and Bocos (n 76).
- 79Software intended for lifestyle or wellness purposes are specifically mentioned as examples of purposes that do not qualify a software as a medical device in Preamble Recital 19 of the MDR. Yu argues the need for regulation of these software applications based on evidence of actual use rather than solely relying on the intended purpose stated by the manufacturer: see Helen Yu, ʻRegulation of Digital Health Technologies in the European Union: Intended versus Actual Useʼ in Carmel Shachar and others (eds), The Future of Medical Device Regulation: Innovation and Protection (Cambridge University Press 2022) 103, 110.
- 80AI-based chatbots for weight loss typically provide users with personalised recommendations and motivational messages, see Han Shi Jocelyn Chew, ʻThe Use of Artificial Intelligence-Based Conversational Agents (Chatbots) for Weight Loss: Scoping Review and Practical Recommendationsʼ (2022) 10 JMIR Medical Informatics e32578 1 <https://doi.org/10.2196/32578>.
- 81As explained in section 3, software running on hardware that is in itself defined as a medical device (eg pacemakers) is deemed an integrated part of the hardware medical device.
- 82Joseph Weizenbaum, Computer Power and Human Reason: From Judgment to Calculation (Freeman 1976), as quoted in Vaidyam and others (n 72).
- 83Kerstin Denecke, Alaa Abd-Alrazaq and Mowafa Househ, ʻArtificial Intelligence for Chatbots in Mental Health: Opportunities and Challengesʼ in Mowafa Househ, Elizabeth Borycki and Andre Kushniruk (eds), Multiple Perspectives on Artificial Intelligence in Healthcare: Opportunities and Challenges (Springer International Publishing 2021) 119, 119-121; Mark Henderson Arnold, ʻTeasing out Artificial Intelligence in Medicine: An Ethical Critique of Artificial Intelligence and Machine Learning in Medicineʼ (2021) 18 Journal of Bioethical Inquiry 121, 122 <https://doi.org/ 10.1007/s11673-020-10080-1>; Liliana Laranjo and others, ʻConversational agents in healthcare: a systematic reviewʼ (2018) 25 Journal of the American Medical Informatics Association 1248, 1256 <https://doi.org/ 10.1093/jamia/ocy072>.
- 84European Commission (n 2), Explanatory Memorandum 2.
- 85Vaidyam and others (n 72).
- 86Denecke, Abd-Alrazaq and Househ (n 83).
- 87Jennifer Nicholas and others, ʻMobile apps for bipolar disorder: a systematic review of features and content qualityʼ (2015) 17 Journal of medical Internet research e4581 <https://doi.org/10 10.2196/jmir.4581>.
- 88Eliane M Boucher and others, ʻArtificially intelligent chatbots in digital mental health interventions: a reviewʼ (2021) 18 Expert Review of Medical Devices 37, 44 <https://doi.org/ 10.1080/17434440.2021.2013200>. Denecke, Abd-Alrazaq and Househ (n 83) 122 also point at chatbotsʼ lack of ability to handle emergency situations as a potential safety risk.
- 89MDCG (n 20) Section 4.2.
- 90Ibid.
- 91Annex VIII MDR, Rule 11, third paragraph.
- 92Article 2(4) MDR.
- 93Annex VIII MDR, Section 2.4.
- 94European Commission, Guidance – MDCG endorsed documents and other guidance <https://health.ec.europa.eu/medical-devices-sector/new-regulations/guidance-mdcg-endorsed-documents-and-other-guidance_en> accessed 29 April 2023.
- 95MDCG (n 20), Annex IV 27.
- 96ibid.
- 97Annex VIII MDR, Section 2.4.
- 98MDCG (n 63) 56.
- 99MDCG (n 20).
- 100Many mental health chatbots available at present are strictly rule-based systems with no AI component. Although they can be used in similar scenarios as AI-based chatbots, they are per definition less autonomous and more predictable.
- 101We discuss possible solutions in Sections 9–10.
- 102Some studies suggest as much as one-third of the workday, depending on clinical role: see Yuliya Pinevich and others, ʻInteraction time with electronic health records: a systematic reviewʼ (2021) 12 Applied clinical informatics 788 <https://doi.org/10.1055/s-0041-1733909>.
- 103David R Kaufman and others, ʻNatural language processing–enabled and conventional data capture methods for input to electronic health records: a comparative usability studyʼ (2016) 4 JMIR medical informatics e35 1 <https://doi.org/ 10.2196/medinform.5544>. On the use of ChatGPT for clinical writing tasks, see also Felipe C Kitamura, ʻChatGPT is shaping the future of medical writing but still requires human judgmentʼ (2023) 307 Radiology e230171 <https://doi.org/10.1148/radiol.230171>.
- 104Tobias Hodgson, Farah Magrabi and Enrico Coiera, ʻEfficiency and safety of speech recognition for documentation in the electronic health recordʼ (2017) 24 Journal of the American Medical Informatics Association 1127, 1129 <https://doi.org/10.1093/jamia/ocx073>.
- 105Errors are common also with manual documentation methods: ibid 1131–1132.
- 106To argue that an EHR documentation system, given that it qualifies as a medical device, falls outside of Rule 11, one would have to contend that the system does not provide the information when it simply transposes or summarises information provided orally by the patient and clinician.
- 107Paragraph 5(a) of Annex III AIA (n 2) mentions ʻAI systems intended to be used by public authorities or on behalf of public authorities to evaluate the eligibility of natural persons for public assistance benefits and servicesʼ. This may potentially cover some medical AI applications. However, the medical AI applications covered by this provision will also be assigned to the ʻhigh-riskʼ category by Rule 11 of Annex VIII MDR, because they are decision support systems.
- 108Konnoth argues that EHR systems, as such, present risks that are similar to risks posed by medical devices, see C Konnoth, ʻAre Electronic Health Records Medical Devices?ʼ in I Glenn Cohen and others (eds), The Future of Medical Device Regulation: Innovation and Protection (Cambridge 2022) 36.
- 109Case C-329/16 SNITEM para 26.
- 110ibid para 33.
- 111It is assumed that conformity to the new requirements introduced by the AIA will be assessed as part of the existing scheme: see Article 43(3) AIA.
- 112Catherine Diaz-Asper and others (n 16).
- 113Palmieri and Goffin (n 4) 12.
- 114Yu (n 79); Palmieri and Goffin (n 4) 11.
- 115Palmieri and Goffin (n 4) 20.
- 116Ibid.
- 117Section 8.